Building Trust in AI: What Leaders Say About User Data and Privacy
Building trust in AI is not just a matter of ethical responsibility; it’s also crucial for the successful adoption and long-term viability of this transformative technology.
But how can organizations navigate the complex ethical landscape surrounding user data and AI training?
In this post, we turn to a panel of business and tech leaders from the Techronicler community, asking them to share their perspectives on the ethical considerations at play and to offer practical guidance on how companies can balance innovation with user privacy.
Their responses provide a roadmap for developing and deploying AI systems that are both powerful and trustworthy.
Read on!
Kirill Bigai
Using user data for AI training raises major ethical concerns, such as consent, transparency, and data security.
Organizations must focus on attaining explicit user consent and effectively communicate how data will be used. At Preply, we ensure user privacy through anonymizing data and focusing on transparency which aligns with global regulations such as GDPR.
Balancing innovation with privacy requires a robust framework, companies should adopt privacy-preserving AI techniques, like federated learning, which trains models without transferring any raw data.
Furthermore, audits and compliance measures conducted on a regular basis can also ensure the ethical use of data.
Lastly, building trust is the key.
Organizations should showcase that innovation does not come at the expense of user privacy, formulating systems where users feel empowered and protected while also benefiting from technological developments.
Ethical AI is not only just a responsibility but it is also a foundation for sustainable innovation.
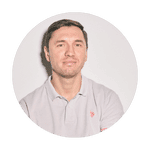
Kirill Bigai
Co-founder, Preply
John Jackson
Transparency and individual control are key to balancing innovation with user privacy.
Companies need to be open about how and why they use data, explaining in clear terms the benefits and how it can fuel innovation as well as the safeguards that are in place to protect individual’s data.
This allows users to make an informed choice about how their data is used and any trade-offs involved in terms of their privacy. It should be coupled with easily accessible tools that give users control over their data, allowing them to decide what is collected and allowing them to opt-out or adjust settings at any time.
Transparency and putting control in the hands of the users builds trust, and in return, research shows that users are more inclined to share their data with companies that prioritise openness.

John Jackson
CEO & founder, Hitprobe
Jason Barnard
The overriding ethical question with AI for any corporation is “do we have the technology, resources and processes that will allow us to reliably tick all the ethical boxes?”
Those ethical boxes include complying with legal standards, obtaining informed consent, respecting data ownership, and anonymizing sensitive information to protect privacy.
Training AI models requires data at a vast scale. As scale increases, control reduces and eventually becomes impossible.

Jason Barnard
CEO, Kalicube
Kevin Baragona
I would mention Opt-In Transparency for training AI models.
The idea is to implement an explicit opt-in process where users actively agree to the use of their data for AI training.
Clearly outline how their data will be used and offer examples of the AI applications it will support. This ensures that users fully understand the implications and can make an informed choice.
According to a study by the University of Michigan, 97% of participants were willing to share their data for AI research if they had control over how it was used.
I suggest companies should also prioritize user privacy by implementing strong data protection measures and regularly updating users on how their data is being used.
This creates a sense of trust and transparency between the company and its users. For instance, Microsoft has recently introduced a new feature in Office 365 that allows users to view and delete their AI training data.
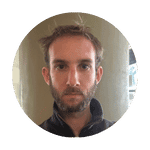
Kevin Baragona
Founder, DeepAI
Ben Michael
When it comes to using user data for AI training, companies first need to think about the legal considerations before anything else.
A lot of the time, the ethical questions they may be pondering will actually be answered by understanding what’s legally required of them.
If you can’t obtain a user’s data through a certain route, for example, then you can’t do it – ethical dilemma solved.
Even when the legality of certain actions are a bit murky, it’s best to err on the side of caution.
Chris Singel
One of the big problems with AI is the ossification or calcification of user data into learning models.
In other words, garbage in, garbage out.
One of the ethical problems of using user data for AI training lies in the fact that users are, in general, terrible. They get stuck in old routines and ways of thinking, don’t follow best security practices, and are prone to oversharing confidential or proprietary information.
Allow AI models to work with you as you train them directly; don’t depend on users to do it for you.
In other words; let the robots do the work!
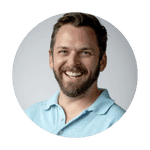
Chris Singel
AI Optimist, Delta Digital
Kate O'Neill
This is very much my area of focus. The challenge isn’t just about privacy policies or data protection — it’s about the precedents we set, and about the opportunity to respect human agency and dignity in an increasingly AI-mediated world.
It’s easy to frame this as a trade-off between innovation and privacy, but we have to treat that as a false dichotomy.
The most innovative and beneficial approaches will be those that enhance both technological capabilities and human autonomy. This requires moving beyond compliance-focused data minimization — although that in itself can be a useful tool in some contexts — toward proactive data stewardship that creates genuine value for users.
The key is not just checking boxes, but helping users understand how their data contributions shape AI development and giving them real choice and control. In other words, transparency and meaningful consent.
Companies that view privacy as an opportunity for building trust rather than a constraint will ultimately develop more sustainable and ethical AI systems.

Kate O’Neill
Founder & Chief Tech Humanist, KO Insights
Deniz Çelikkaya
One of the key ethical challenges lies in informed consent—users often aren’t fully aware their data is being used to train AI, raising concerns about transparency and trust.
Additionally, there’s the risk of reinforcing biases or exposing sensitive information if datasets aren’t properly anonymised.
To balance innovation with privacy, companies must adopt a privacy-by-design approach, integrating data protection at every stage of AI development.
This includes minimising data collection, ensuring robust anonymisation, and implementing differential privacy techniques. Regulatory compliance, such as adherence to GDPR or similar frameworks, is also critical.
Innovative solutions, like synthetic data, can further drive AI advancements while reducing reliance on personal data.
Striking this balance not only safeguards users but also builds trust, ultimately fostering more sustainable innovation.
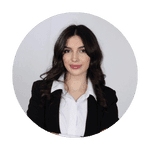
Deniz Çelikkaya
Founder, Atka
Neil Sahota
This is a delicate balance between innovation and responsibility.
First, privacy concerns dominate the conversation. Users expect transparency and consent when their data is collected and monetized, but companies often need help communicating their uses clearly. Data misuse or unauthorized sharing erodes trust and leads to legal repercussions.
Second, bias in datasets is a critical issue. When companies fail to ensure diversity in their data, AI systems will perpetuate or even amplify skewed views and societal inequalities, affecting everything from hiring decisions to healthcare access.
The accountability question looms large in conjunction: who takes responsibility when AI-driven decisions cause harm? Companies must actively design safeguards to prevent damage and mitigate risks.
Finally, many organizations grapple with the temptation to prioritize profits over user rights. Ethical AI demands proactive action, which means embedding fairness, security, and inclusivity into the heart of AI development strategies.
The key is to embed ethical principles into every stage of the innovation process. Any organization should actively adopt privacy-by-design frameworks to ensure privacy safeguards are built into products and systems from the beginning rather than being an afterthought.
Transparent data practices are critical. Organizations must clearly communicate how they collect, store, and use data so that users make informed choices.
Additionally, organizations should Invest in robust data anonymization techniques. This allows them to extract valuable insights while protecting individual identities. They should also regularly audit and refine their algorithms to mitigate risks and unintended consequences.
This requires broad collaboration. Engaging ethicists, legal experts, and diverse stakeholders will foster a broader perspective on responsible innovation.
Furthermore, organizations must go beyond compliance by cultivating a culture of accountability.
Leaders should champion privacy as a core value and demonstrate that ethical innovation is a competitive advantage in building trust and long-term success.
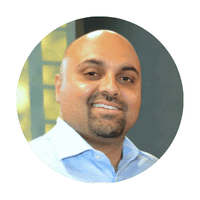
Neil Sahota
CEO, ACSI Labs
Anbang Xu
I think the ethical debate around using user data for AI training is often framed too narrowly. It’s not just about privacy versus innovation—it’s about control and consent.
If users feel ownership of their data and are actively part of the decision-making process, companies can foster trust while still innovating.
Imagine AI systems where users can choose what data to share, with clear benefits outlined upfront—this shifts the conversation from “Are we overstepping?” to “How can we collaborate?”
One innovative strategy is embedding data controls directly into AI platforms, akin to a privacy dashboard. Users could toggle permissions in real-time, deciding how much of their data contributes to training.
Federated learning is a step in the right direction, but even it could go further by giving users tangible incentives—like enhanced AI performance tailored to their preferences.
I think the companies that win this race won’t just check legal boxes but redefine data ethics as a partnership. That approach isn’t just innovative—it’s future-proof.
On behalf of the Techronicler community of readers, we thank these leaders and experts for taking the time to share valuable insights that stem from years of experience and in-depth expertise in their respective niches.
If you wish to showcase your experience and expertise, participate in industry-leading discussions, and add visibility and impact to your personal brand and business, get in touch with the Techronicler team to feature in our fast-growing publication.