Transparency and Consent: Ethical Innovation for AI and User Data
The development of powerful AI technologies is pushing the boundaries of what’s possible, but it’s also creating a pressing need for clear ethical guidelines.
A central point of concern is the use of user data to train AI models, a practice that can offer significant benefits but also carries inherent risks to privacy and security.
Recent discussions surrounding Microsoft’s AI training practices have highlighted this issue.
To shed light on the ethical considerations at play, we reached out to the Techronicler community of tech leaders to share their perspectives.
This post reveals some of their insights, exploring how companies can balance the drive for AI innovation with the imperative to protect user privacy and build trust.
The first insight from Dr. Ryan Ries, AI and Data Scientist, also helps us understand how LLMs work.
Read on!
Dr. Ryan Ries
A lot of people still don’t understand how LLMs work – they are still convinced that the system is thinking and learning and understanding their business.
At the end of the day, an LLM is just creating vectors for the data and then doing a prediction of what answer you would get given the input question.
It is a complete mathematical solution.
When you take and add more data to the system, you are just making concepts and word association connections. The more data, the easier it is to predict the solution.
99.9% of all user data has no real proprietary impact. That .1% is then buried in a massive amount of vector space as a concept, so the probabilities are fairly small that you will expose anything of relevance.
Of course, some university researchers have proven in small models that you can rebuild the training data set, but that was only because they knew what to look for.
At the end of the day, due to the size of the vector space and predictive nature of LLMs, it really isn’t that big of a deal to train on users data.
Of course, you should be sure to ask for permission before doing it, but as far as harming a company, the likelihood is much smaller from an LLM than it is from some phishing attack and an employee giving away too much information.
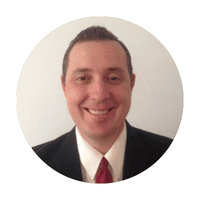
Dr. Ryan Ries
Chief AI and Data Scientist, Mission Cloud
Jeff Fryer
As a Fractional CMO, I see the ethical challenge of using user data for AI training as a matter of trust versus risk.
Customers don’t just hand over data—they expect companies to respect and protect it. The moment that trust breaks, you’re not just facing legal challenges; you’re damaging your brand equity.
Balancing innovation with privacy starts with clarity and consent.
Be upfront about what data you’re using, why it matters, and what users get in return. It’s not just about limiting data collection; it’s about showing restraint in how it’s applied.
Companies need leadership that ensures ethical practices aren’t an afterthought but baked into their AI strategies from day one.
Ultimately, treating data like a privilege, not a right, is the way forward. Ethical AI isn’t just the responsible approach—it’s how companies earn loyalty and competitive advantage in the long run.
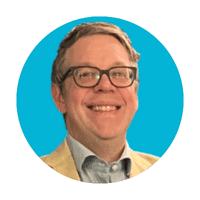
Jeff Fryer
Founder & CEO, J+ Advisors
Neil Sahota
There exists a delicate balance between innovation and responsibility.
First, privacy concerns dominate the conversation. Users expect transparency and consent when their data is collected and monetized, but companies often need help communicating their uses clearly. Data misuse or unauthorized sharing erodes trust and leads to legal repercussions.
Second, bias in datasets is a critical issue. When companies fail to ensure diversity in their data, AI systems will perpetuate or even amplify skewed views and societal inequalities, affecting everything from hiring decisions to healthcare access.
The accountability question looms large in conjunction: who takes responsibility when AI-driven decisions cause harm? Companies must actively design safeguards to prevent damage and mitigate risks.
Finally, many organizations grapple with the temptation to prioritize profits over user rights. Ethical AI demands proactive action, which means embedding fairness, security, and inclusivity into the heart of AI development strategies.
The key to ensuring responsible innovation without compromising privacy is to embed ethical principles into every stage of the innovation process.
Any organization should actively adopt privacy-by-design frameworks to ensure privacy safeguards are built into products and systems from the beginning rather than being an afterthought.
Transparent data practices are critical. Organizations must clearly communicate how they collect, store, and use data so that users make informed choices.
Additionally, organizations should Invest in robust data anonymization techniques. This allows them to extract valuable insights while protecting individual identities. They should also regularly audit and refine their algorithms to mitigate risks and unintended consequences. This requires broad collaboration.
Engaging ethicists, legal experts, and diverse stakeholders will foster a broader perspective on responsible innovation.
Furthermore, organizations must go beyond compliance by cultivating a culture of accountability.
Leaders should champion privacy as a core value and demonstrate that ethical innovation is a competitive advantage in building trust and long-term success.
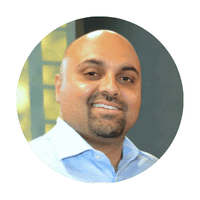
Neil Sahota
United Nations AI Advisor and CEO, ACSILabs.
Neda Nia
The ethical use of user data for AI training is a key concern for enterprise CISOs and data privacy teams.
As requirements evolve, tools, processes, and governance – all play a critical role in ensuring ethical lines are not crossed and users’ trust is not compromised.
To balance innovation (the speed and ambition) and privacy, businesses should define what constitutes sensitive, proprietary, and generic data. Clear data labeling helps prevent the unintended use of sensitive data during AI model training, ensuring privacy throughout the data lifecycle and governing it through enterprises value chain.
Privacy-preserving techniques like data anonymization and differential privacy enable AI models to learn from data (and the logic of it) while safeguarding user identities. These methods ensure data logic is used without exposing individuals.
While AI ethics remain a developing field, companies can protect privacy by adopting transparent policies, governance frameworks such as ISO 27001/2, and advanced privacy technologies—all while driving AI-powered innovation.

Neda Nia
Chief Product Officer, Stibo Systems
Andy Gillin
The ethical use of user data in AI training hinges on transparency and consent.
Companies must clearly communicate how they use data and ensure users consent to their information being part of AI training.
Privacy can be maintained and innovation encouraged through a framework called “differential privacy.” This technique ensures that individual user information remains anonymous and unaffected even when used for model training.
Companies should prioritize encrypting data to protect user identities, while still benefiting from the aggregate information.
Being upfront with users about these methods builds trust, ensuring they understand that while their data contributes to development, their personal details remain confidential.
Using regular audits and transparent privacy practices can help companies maintain this balance, keeping both innovation and trust intact.
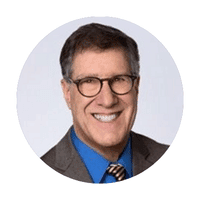
Andy Gillin
Attorney & Managing Partner, GJEL Accident Attorneys
Karan Singh Bhakuni
One of the biggest challenges when it comes to AI is balancing innovation with user privacy. Companies need to be really mindful about how they collect and use user data.
In my experience, getting clear, explicit consent from users is key – they should know what data is being collected and how it’s going to be used.
Another important step is anonymizing data whenever possible, to ensure privacy while still using data effectively.
Ultimately, transparency and trust are the foundation here.
Companies that prioritize clear communication, build ethical AI practices, and respect user privacy will not only avoid potential risks but also strengthen user confidence.
Aaron Fulkerson
At its core, the dilemma lies in balancing the value of innovation with the imperative to respect data privacy and sovereignty.
Users must clearly understand how their data is leveraged, and companies must prioritize transparency to build trust.
Techniques like anonymization have long been the backbone of data sharing in industries like high tech, financial services, insurance, healthcare and many others; however, these methods are increasingly vulnerable to deanonymization attacks.
With LLMs in widespread use, the risks are magnified.
As such, data anonymization and similar strategies have become essentially obsolete and thus new, more robust safeguards are essential to prevent misuse.
Confidential computing ensures data sovereignty while seamlessly enabling scalable AI adoption, a necessity for businesses that want to stay competitive and innovate safely.
The big players, Amazon, Microsoft, Google, NVIDIA, AMD, and Apple, are doubling down on confidential computing, rolling out capabilities to make it easier for companies to protect their data and models, and prioritize data sovereignty and user privacy in the process.
Elle Farrell-Kingsley
Interestingly, the allegation of Microsoft using Office documents for AI training highlights potential legal violations beyond ethical concerns.
The General Data Protection Regulation (GDPR) in the EU mandates transparency (Article 5) and lawfulness of processing (Article 6), meaning data cannot be repurposed for AI training without explicit consent. In the US, the California Consumer Privacy Act (CCPA) and California Privacy Rights Act (CPRA) require clear disclosure of data use and provide opt-out rights.
At the same time, the Federal Trade Commission Act (FTC Act) prohibits deceptive practices, including misleading claims about data handling. If companies bypass these regulations, they risk fines and reputational damage.
Ethically, even with legal compliance, using personal data without explicit user understanding undermines trust.
Companies must consider the duty of care in protecting user data, and integrating privacy-preserving techniques like federated learning while fostering transparent, respectful engagement with users.
With so many competitors eager to take its place, if the allegations were later found to be true, Microsoft would be putting itself in a particularly compromising position.

Elle Farrell-Kingsley
Presenter, Researcher Advisor, Author, Elle F. Kingsley
Peter Smith IV
Microsoft’s recent denial about using Office documents for AI training shines a light on the ethical tightrope companies walk as they develop increasingly sophisticated artificial intelligence (AI) systems.
While AI promises to revolutionize everything from healthcare to transportation, its reliance on vast amounts of user data raises critical questions about privacy and responsible development.
The central ethical dilemma lies in balancing the potential benefits of AI with the need to protect user information. Companies eager to leverage data for AI training must prioritize transparency and user consent.
This means clearly informing users how their data might be used and providing a mechanism to opt out.
Furthermore, even with consent, companies have an ethical obligation to anonymize data whenever possible and implement robust security measures to prevent breaches. AI models should be regularly audited for bias to ensure fairness and avoid discriminatory outcomes.
Ultimately, building trust is paramount. By prioritizing ethical data handling practices, companies can foster an environment where AI innovation flourishes alongside user privacy.
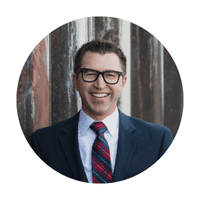
Peter Smith IV
Business attorney and Co-founder, Smith + Malek
Ryan Farley
I think you start to see some extra ethical concerns when you are using potentially personal or private communication, information, or creative projects to train your AI models.
If your company does have access to this type of client data, then I do think there’s a responsibility there to allow clients to opt in to AI training, but not make this the standard.
Allowing users to opt in tends to be a good way to go about this and navigate ethical concerns no matter your industry or what type of data you have access to.
On behalf of the Techronicler community of readers, we thank these leaders and experts for taking the time to share valuable insights that stem from years of experience and in-depth expertise in their respective niches.
If you wish to showcase your experience and expertise, participate in industry-leading discussions, and add visibility and impact to your personal brand and business, get in touch with the Techronicler team to feature in our fast-growing publication.